Projects
5 minutes
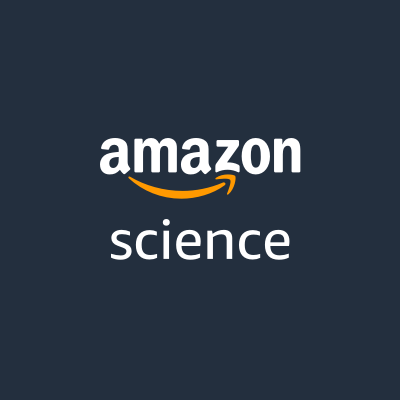
PCo3D: Physically Plausible Controllable 3D Generative Models
Amazon Research Award, PI, with Aleš Leonardis
Generative AI has shown remarkable performance across various applications involving content generation, showcasing its potential in both academic research and industrial settings. While its effectiveness in generating images and videos is well-established, there exists a notable gap when it comes to 3D content creation, particularly in the consideration of physical properties during the generation process. Another gap is the controllability of the physics-aware generation. In this research, we aim to make a step forward towards bridging the gaps.
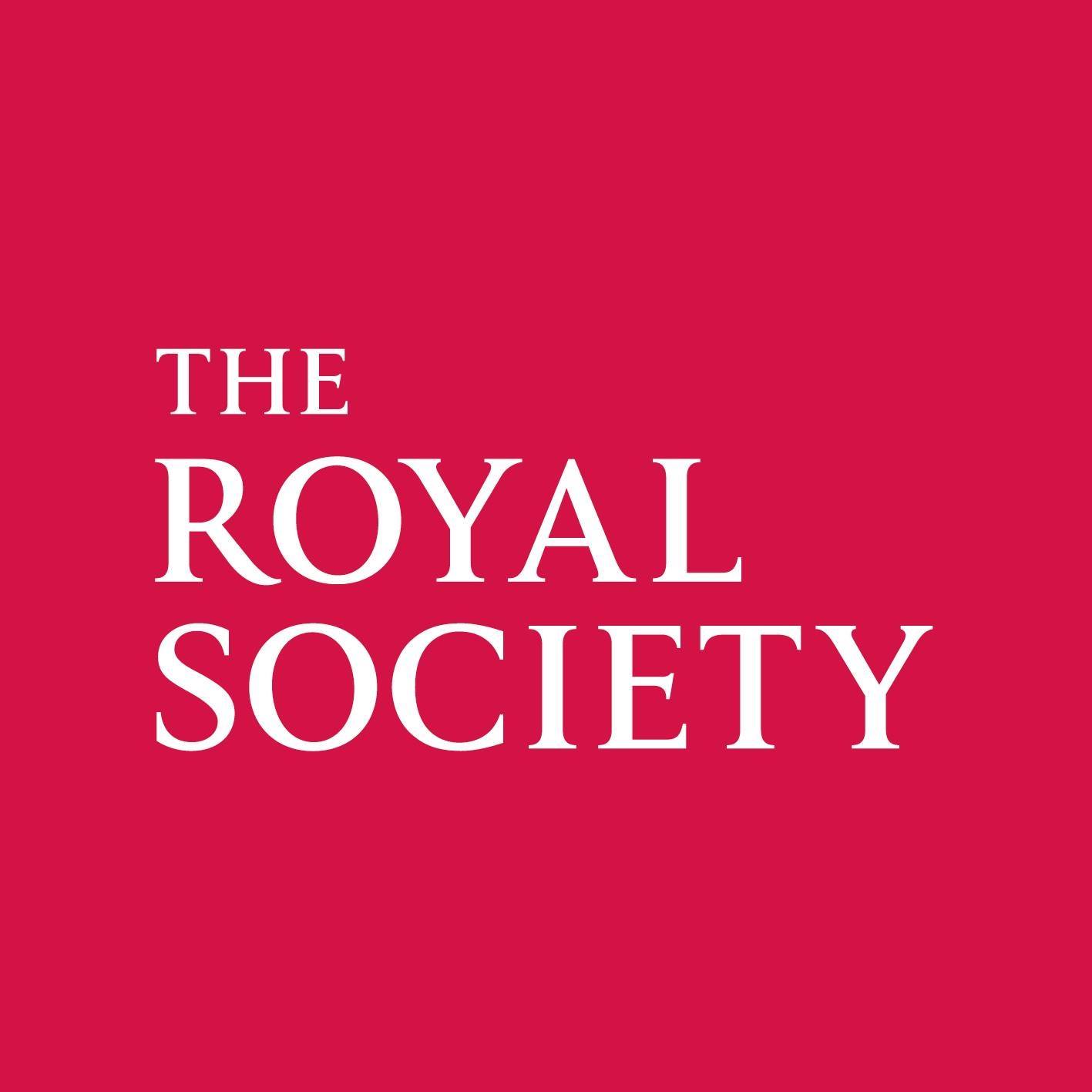
CLRM3D: Continual Large-scale Representation Learning from Multi-Modal Medical Data
The Royal Society, PI
Medical data (e.g. CT, MRI, ultrasound, clinical reports) plays a key role in clinical diagnosis and analysis due to its bridging role between clinicians and patients. Doctors and clinicians have been deriving experience-based analytical approaches for domain-specific clinical diagnosis after years of data observation and training. Recent advances in machine learning (ML) showed the possibility of diagnosis automation. Training of such models heavily depends on expert manual annotations. A model trained on one specific dataset cannot generalise well to new data. This research aims to use ML algorithms develop automated solutions for unsupervised continual learning from open-world healthcare data.
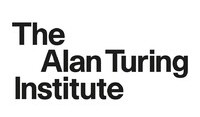
Holistic Hateful Video Detection and Localisation via Multi-Modal Graph Learning
The Alan Turing Institute, Co-I, with Zeyu Fu
Social media companies like YouTube and Facebook employ human moderators to review user-flagged videos before they escalate and cause long-term harm to society. However, given the sheer volume of daily uploads, ensuring compliance with established policies becomes challenging. Smaller platforms with limited resources may struggle to afford human moderators, thereby making affordable and automated hateful content detection solutions highly desirable. Current automated approaches mainly rely on textual media or features for identifying hateful content, with fewer studies focused on the analysis of videos. This research domain presents its distinct set of challenges. In this project, we will try to alliviate the challenges via multi-modal graph learning.
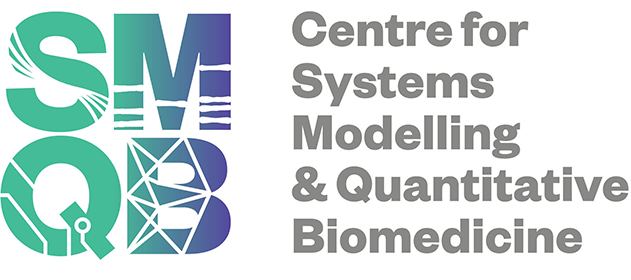
Directed forgetting in human brains and artificial neural networks
Centre for Systems Modelling & Quantitative Biomedicine, Co-I, with Paul Muhle-Karbe
This project will examine how humans and artificial neural networks (ANNs) remove information from memory. In daily life, forgetting can be frustrating but is vital for prioritising the most relevant information. At present, the mechanisms that underpin directed forgetting are challenging to infer, as they are implemented in neural circuits at a fine spatial scale that cannot be resolved with human brain imaging. To address this challenge, we will delineate the mechanisms by which ANNs form and remove memories for effective task performance to simulate mechanisms within the human brain and generate testable new predictions for future research.
Finished:
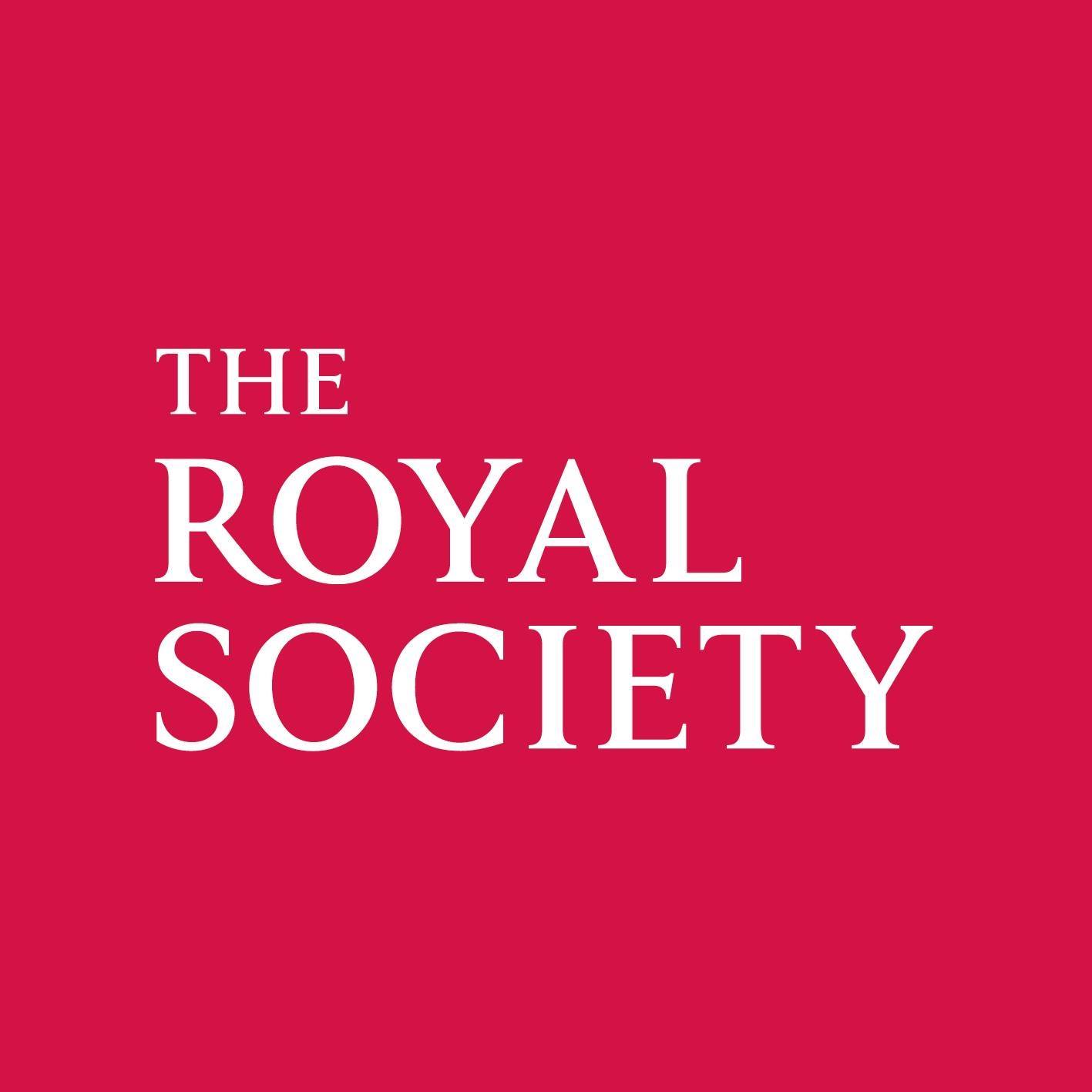
COMPaD: Commercial-Oriented Multi-modal Poster Generation and Design
The Royal Society, PI
The poster design market for commercial users has long thrived, but traditional user-designer collaboration often suffers from time-consuming and inefficient communication, resulting in compromised designs. This creates a pressing need for an automated and user-friendly solution for commercial poster generation. Recent advancements in artificial intelligence (AI) have shown great promise in generating high-quality content. In this collaborative research, we aim to make a step forward towards bridging this gap.
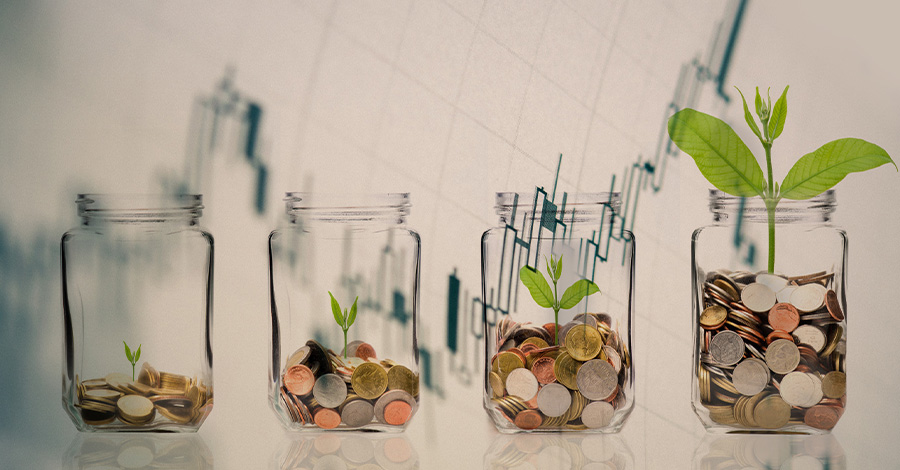
Bridging Human Brain Electrophysiology and Artificial Neural Network to Understand How Visual Representations Emerge
Institute for Interdisciplinary Data Science and AI (IIDSAI), PI, with Ole Jensen
Understanding how the human brain works is a longstanding and challenging question. Inspired by human neuroscience, artificial neural networks (ANNs) have shown remarkable progress across various fields. This proposed research aims to study the relationship between human brain and ANNs, from the perspective of visual representations (e.g. recognition, detection), with a particular focus on sequential dynamic data and visual memory.
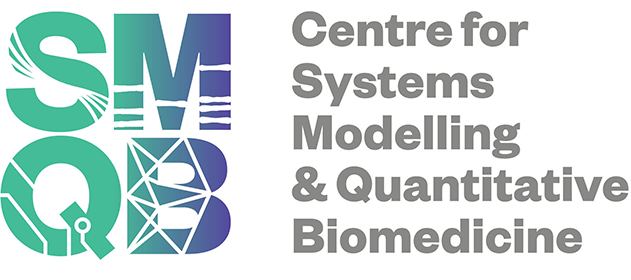
Visual Dynamics in Human Brain and Artificial Neural Network
Centre for Systems Modelling & Quantitative Biomedicine, PI, with Ole Jensen
This proposed research aims to study the differences and relationships between the human brain and artificial neural networks (ANNs) in terms of spatiotemporal dynamics. Specifically, we will look into how time activations present in human brains and ANNs given sequences of visual data for recognition. This research will also investigate how new categories form in the human brain and ANNs in a dynamic manner. We will try to answer if the working mechanisms behind human brains are similar to what an ANN does, and following that leverage what we learn from human brains to help the design of ANNs.
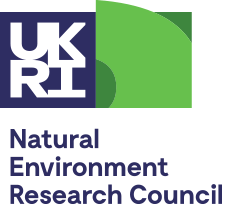